Machine learning H2 storage properties of high entropy alloys. Towards designer materials with specific thermodynamic properties.
Research question: Can ML models predict and direct the synthesis of high entropy alloys with targeted thermodynamic stability?
References: DOI: 10.26434/chemrxiv.13697464
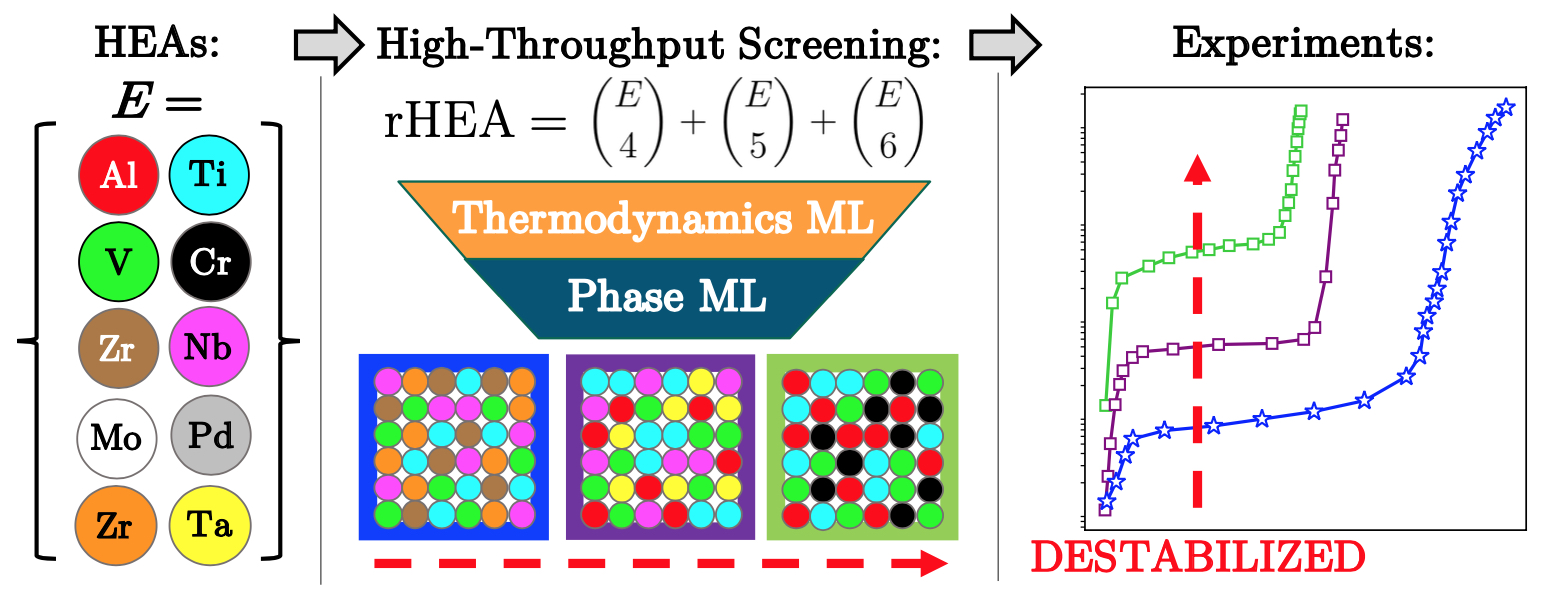
Nanoporous materials for optimal gas storage. Stat mech models elucidate theoretical performance limits.
Research question: What is the gas deliverable capacity limit in intrinsically flexible nanoporous materials?
References: DOI: 10.1039/D0ME00122H
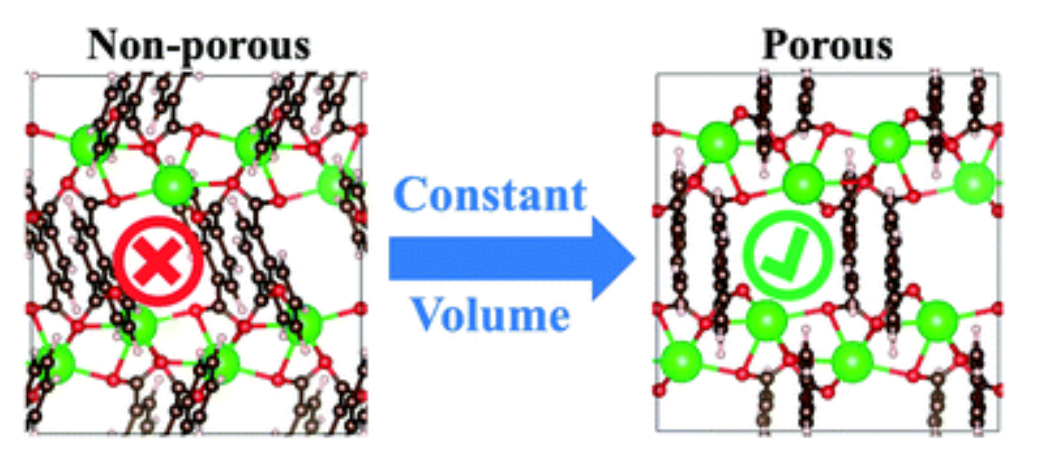
Machine learning an empirical metal hydride design rule. Targeted discovery of energy storage materials.
Research question: Can machine learning insights of intermetallic hydride thermodynamics aid in the design of novel hydrogen storage materials?
References: DOI: 10.1021/acs.jpclett.9b02971
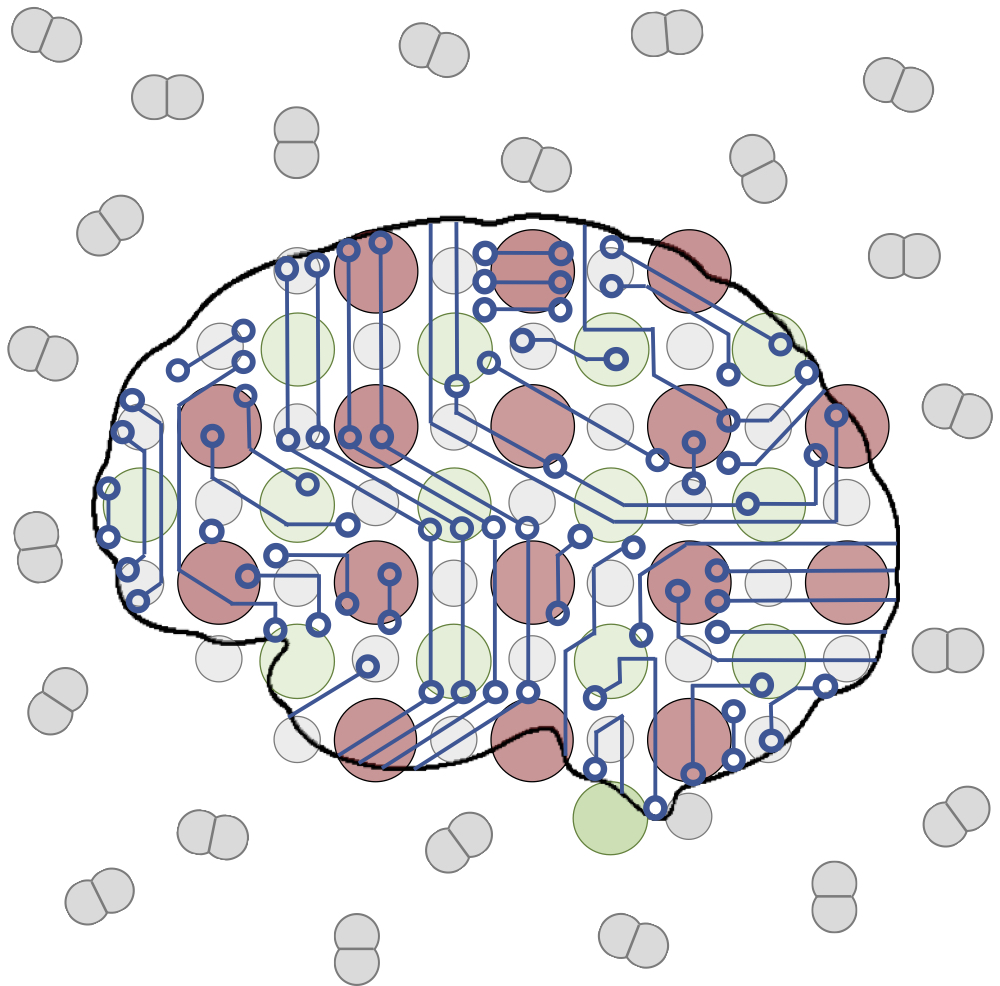
Molecular dynamics and Transition Matrix Monte Carlo. Combining simulation techniques for unconstrained adsorption simulations.
Research question: Can we combine molecular simulation techniques in novel ways to efficiently predict thermodynamic adsorption properties of non-rigid porous materials?
References: DOI: 10.1021/acs.jpclett.9b02449
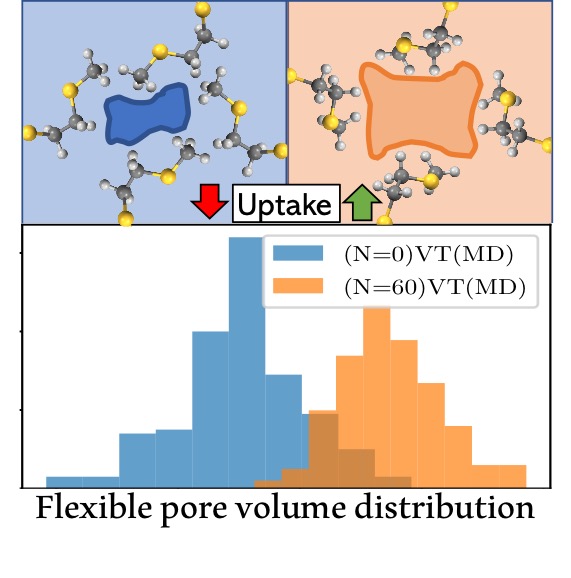
Reinforcement learning and plasma medicine. Sim-to-real transfer reinforcement learning for the control of cold atomospheric plasma.
Research question: How can we leverage sim-to-real transfer learning techniques to develop a robust controller for an atmoshpheric pressure plasma jet using only simulated data?
References: DOI: 10.1088/1361-6595/ab3c15
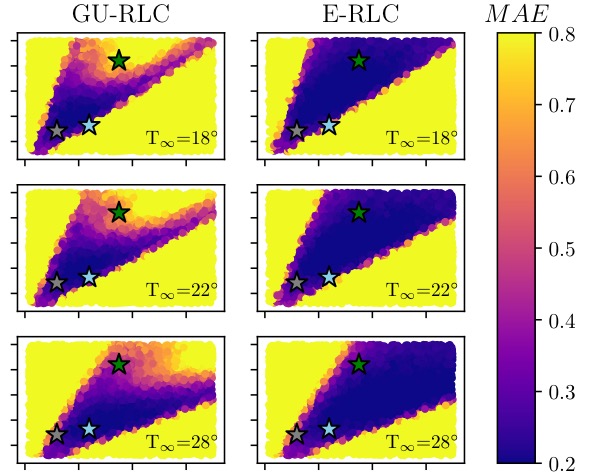
Flat-histogram Monte Carlo simulations. Improved algorithms for predicting materials' performance.
Research question: Can we utilize enhanced Monte Carlo sampling and other statistical mechanics techniques to more effectively identify promising materials for chemical separations via computer simulations?
References: DOI: 10.1021/acs.jctc.8b00534
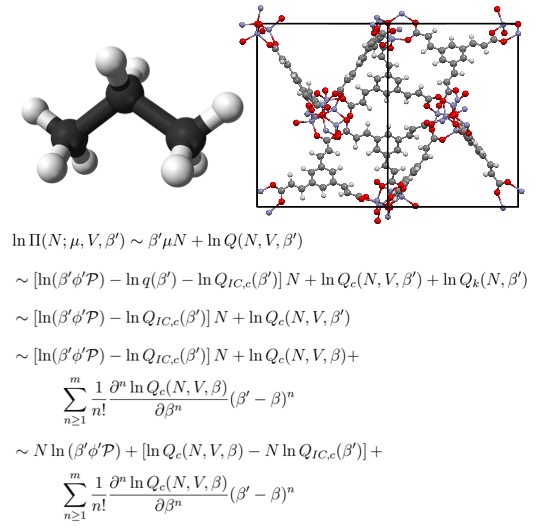
Neural network potentials for molecular simulation. Machine learning the potential energy surfaces of condensed matter systems.
Research question: How well can neural networks approximate quantum chemical forces and predict important thermodynamic and kinetic properties of crystalline materials?
References: Coming soon
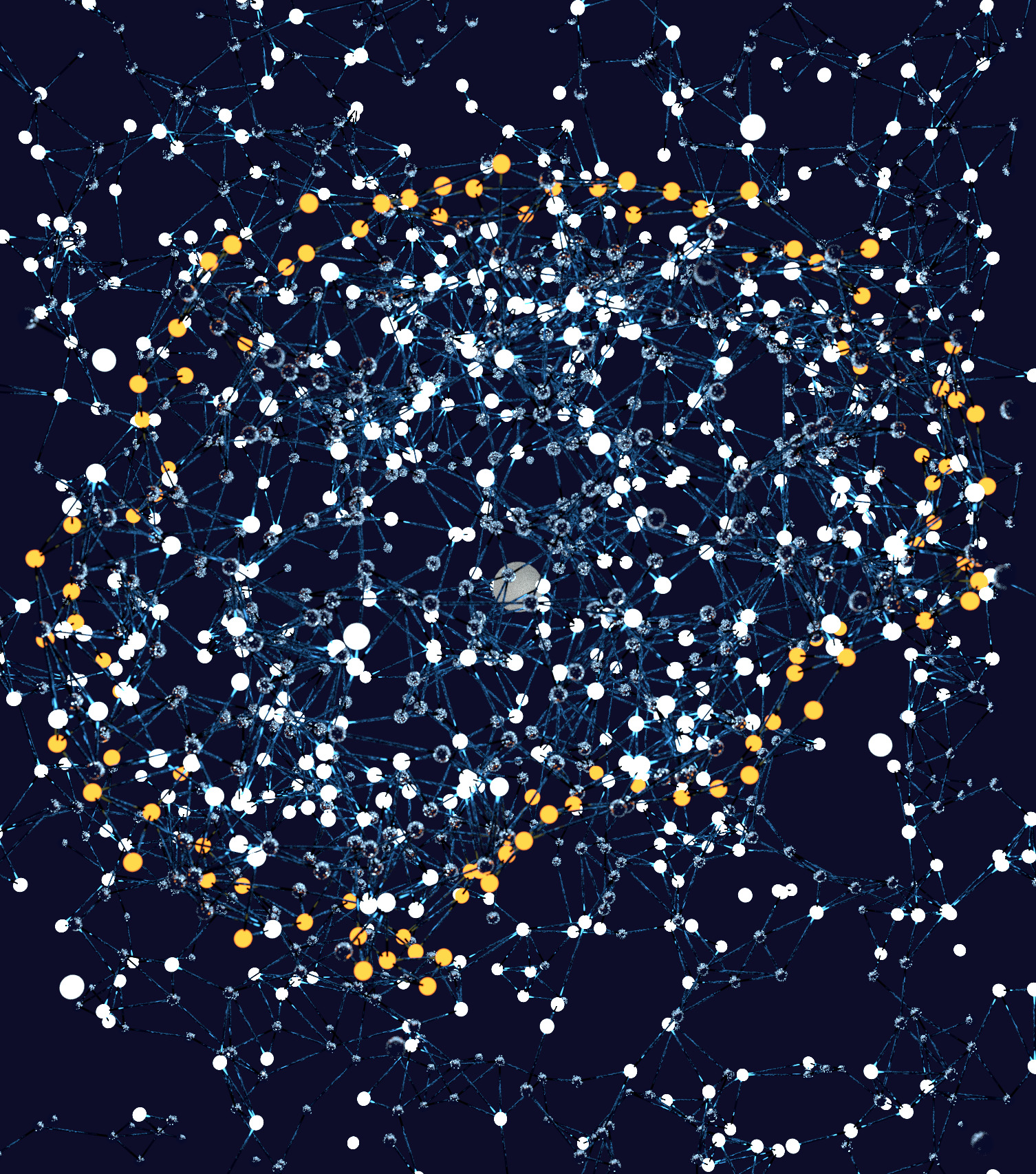
Minimum cut graph theory. Crystal surface generation and predicting 2D zeolites.
Research question: Can a graph theory formalized approach to crystal surface generation yield information of preferred crystal faces and help predict which crystalline materials may be likely to exhibit 2D forms?
References: DOI: 10.1021/acscentsci.7b00555
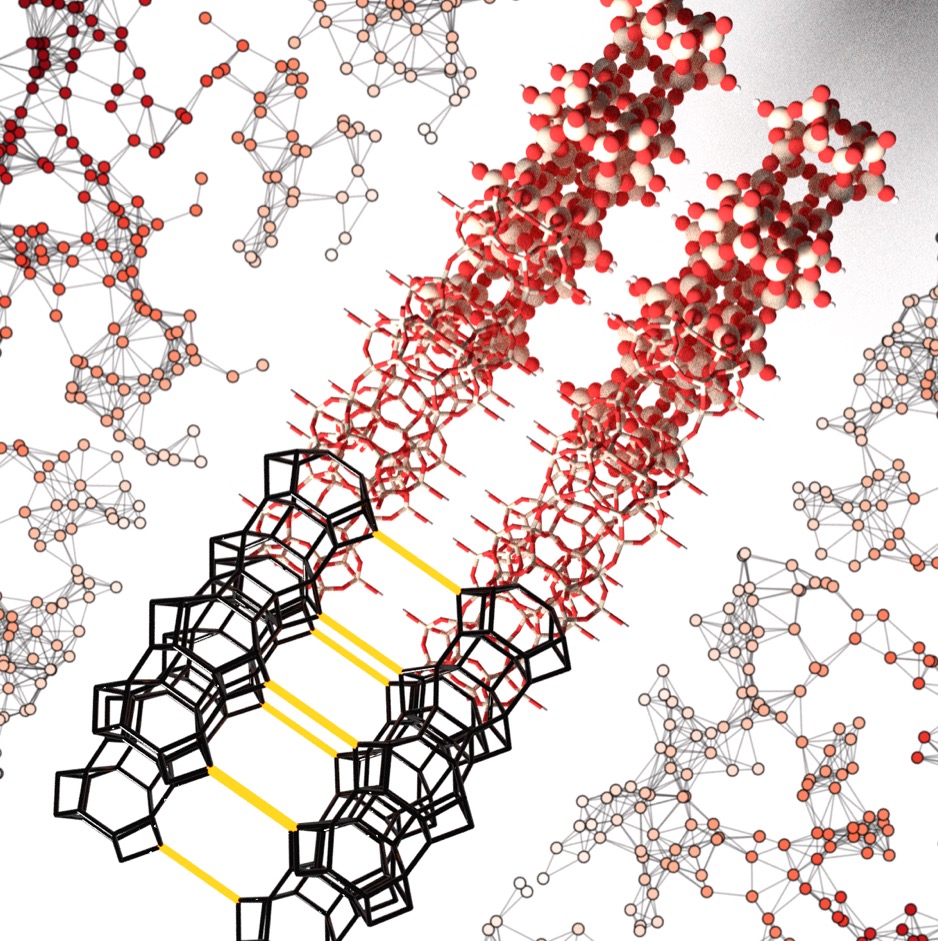
High-throughput materials screening. Understanding the interplay of flexibility and adsorption.
Research question: Does the assumption of static crystal structures bias the result of high-throughput screening predictions of adsorption behavior?
References: DOI: 10.1021/jacs.7b01688
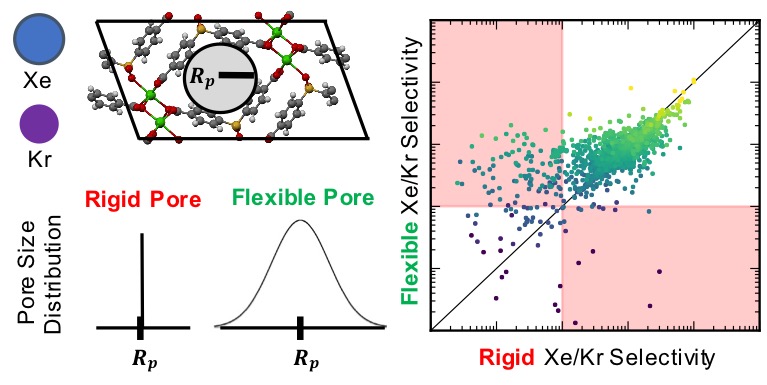
Cheminformatics and computer vision algorithms. In silico prediction of novel porous crystals.
Research question: Can we design an algorithm to hypothetically enumerate a special class of crystal structures (known as 1D rod materials) and are any of them experimentally synthesizable?
References: DOI: 10.1039/C6SC01477A , DOI: 10.1021/acs.jpcc.6b10363
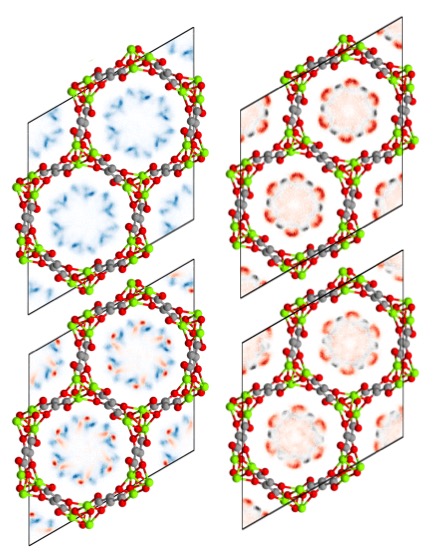
Converting scientific artwork into coloring book images. Art that promotes science for all ages.
Research question: What is the best way to convert an image into a black and white trace that can be used for a coloring book?
References: ColorBooked code; Blog post
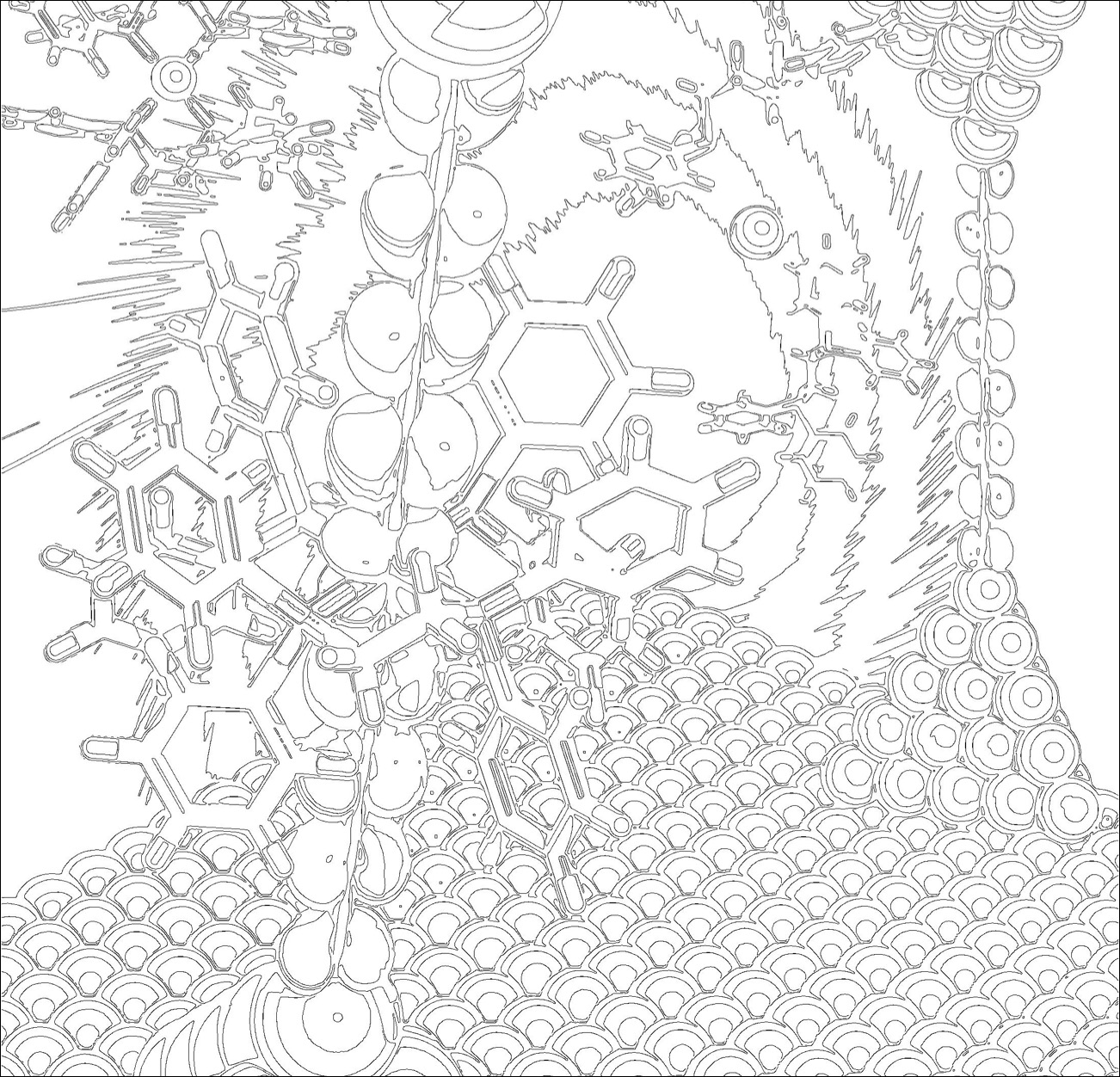